SimilarityIndex™ | Markets Report
The foundation of an evidence-based strategy is an accurate benchmark. The SimilarityIndex™ is a new set of benchmarks generated from the health economy’s first machine-learning-based SimilarityEngine™.
- Introduction
- Demand
- Supply
- Yield
- Conclusion
- Methodology
Report Summary
As a society, we have been primed to determine what is “best” using third-party rankings and abstract measures. What are the top five restaurants in New York? What are the best colleges in the country? Healthcare is no exception. What are the best hospitals in America? Who are the best orthopedic surgeons in Tampa?
In healthcare, these lists often factor in self-reported, survey-based data that are misaligned with patient outcomes. As a result, the entire health economy pursues aspirational and arbitrary benchmarks to guide critical decisions from M&A to value-based network development.
This report is intended as a companion to SimilarityIndex™ | Markets, the industry’s first set of benchmarks that uses machine learning to analyze the similarity of U.S. markets. With data-driven benchmarks, health economy stakeholders – from policymakers and health plans to providers and life sciences – can form evidence-based strategies to navigate the increasingly complex health economy.
Key Takeaways
- Healthcare is inherently local, and therefore a complete understanding of a market across multiple factors is a requirement for meeting the care needs and preferences of individual patient populations.
- Although CommonSpirit, Ascension and Trinity Health are considered peers by revenue and operating structure (i.e., non-profit), their “top” markets are not all peers in terms of market concentration.
- Similarity on one or even two metrics (e.g., operating structure and revenue) does not always correlate with similarity across all other metrics.
Methodology
A variety of data sources were leveraged as part of this research, with most insights gleaned from proprietary Trilliant Health datasets: all-payer claims, consumer, provider directory, and demand forecast. Trilliant Health’s all-payer claims database combines commercial, Medicare Advantage, traditional Medicare, and Medicaid claims representing more than 320M Americans. The analytics were calculated using a variety of methods, and the projections were created using machine learning. Additional data was gleaned from sources such as the U.S. Census Bureau and the National Vital Statistics Program.
The SimilarityEngine™ was developed using a machine-learning-based model that applies the mathematical concepts of “cosine similarity.” Cosine similarity measures the cosine of the angle between two vectors projected in a multi-dimensional space. If the angle between the two vectors is 90 degrees, the cosine similarity will have a value of 0, meaning that the two vectors are perpendicular to each other denoting no correlation between them. The closer the cosine value is to 1, the more similar the vectors.
About the Author
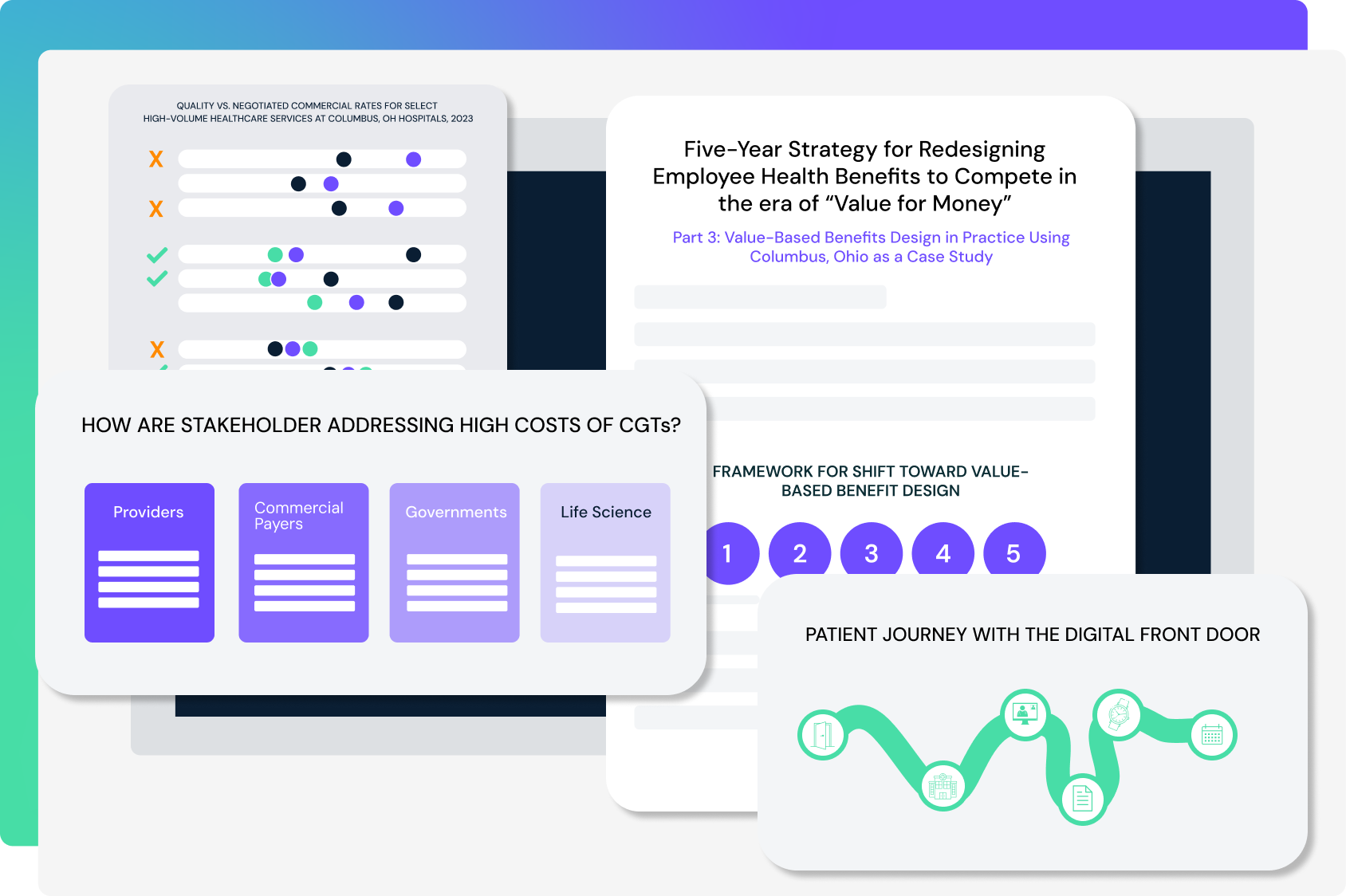
Compass+
Track the latest developments across the health economy.
Subscribe to Compass+ for additional analyses, market-specific trends, ongoing tracking throughout the year and more. With a subscription, you will also receive:
- Tailored analysis and health policy insights
- Interactive data dashboards to track trends
- Executive commentary and perspectives
- A slide library to inform internal presentations